Critical Spatial Data Science (or Geographic Data Science) analyses quantitative data with some form of spatial identifier – for example, a coordinate, a street name, or a census block – to generate new knowledge.
In carrying out analysis of spatial data, a critical analysis is typically underpinned by theories that help us to understand – and therefore to best represent – complex real-world processes. Critical Spatial Data Science has wide-ranging applications with the potential to provide new insights into the distribution and dynamics of populations and societies across space and time. However, most commonly it seeks to understand and evidence socio-spatial inequalities, for example, inequalities in health, infrastructure, or education. Critical Spatial Data Science is founded on principles of openness, transparency, and reproducibility. At its best, the approach can be used to evidence and challenge injustice and have real-world impacts beyond academia. The field is rapidly expanding, drawing on an increasingly diverse range of spatial methods and data.
Download the Critical Spatial Data Science ‘how to’ guide.
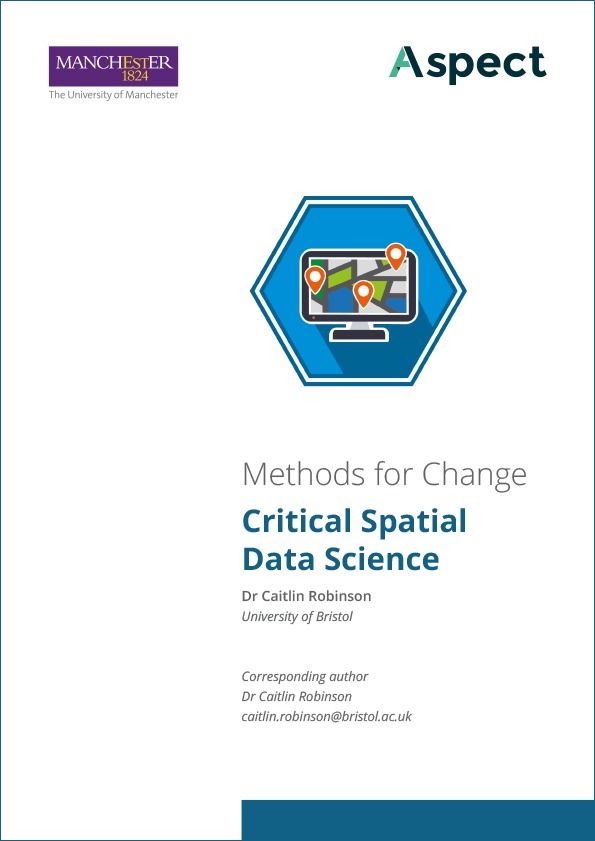
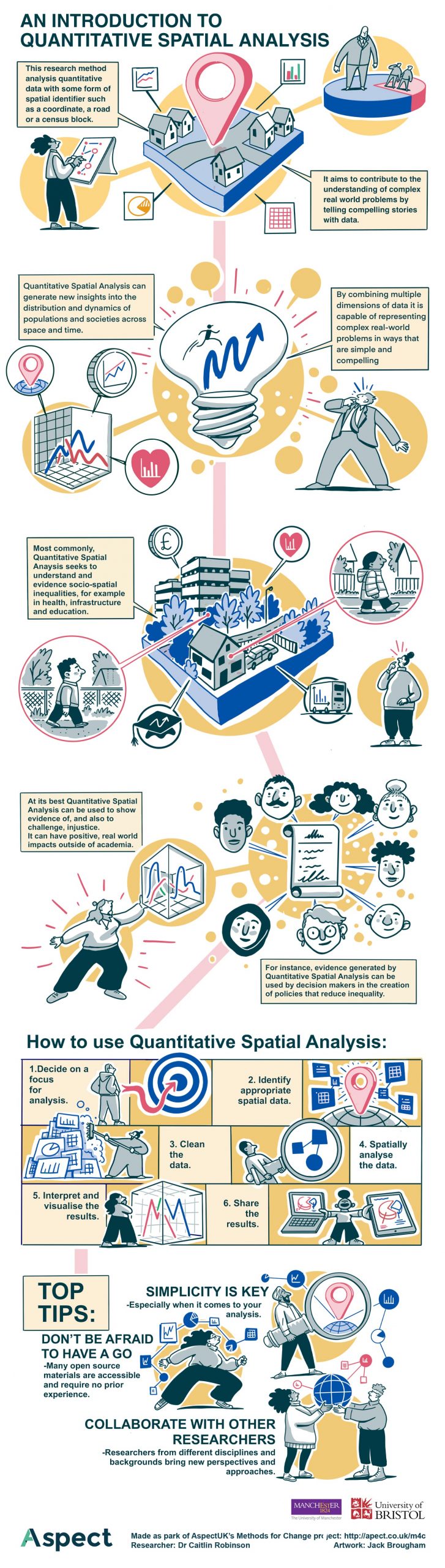